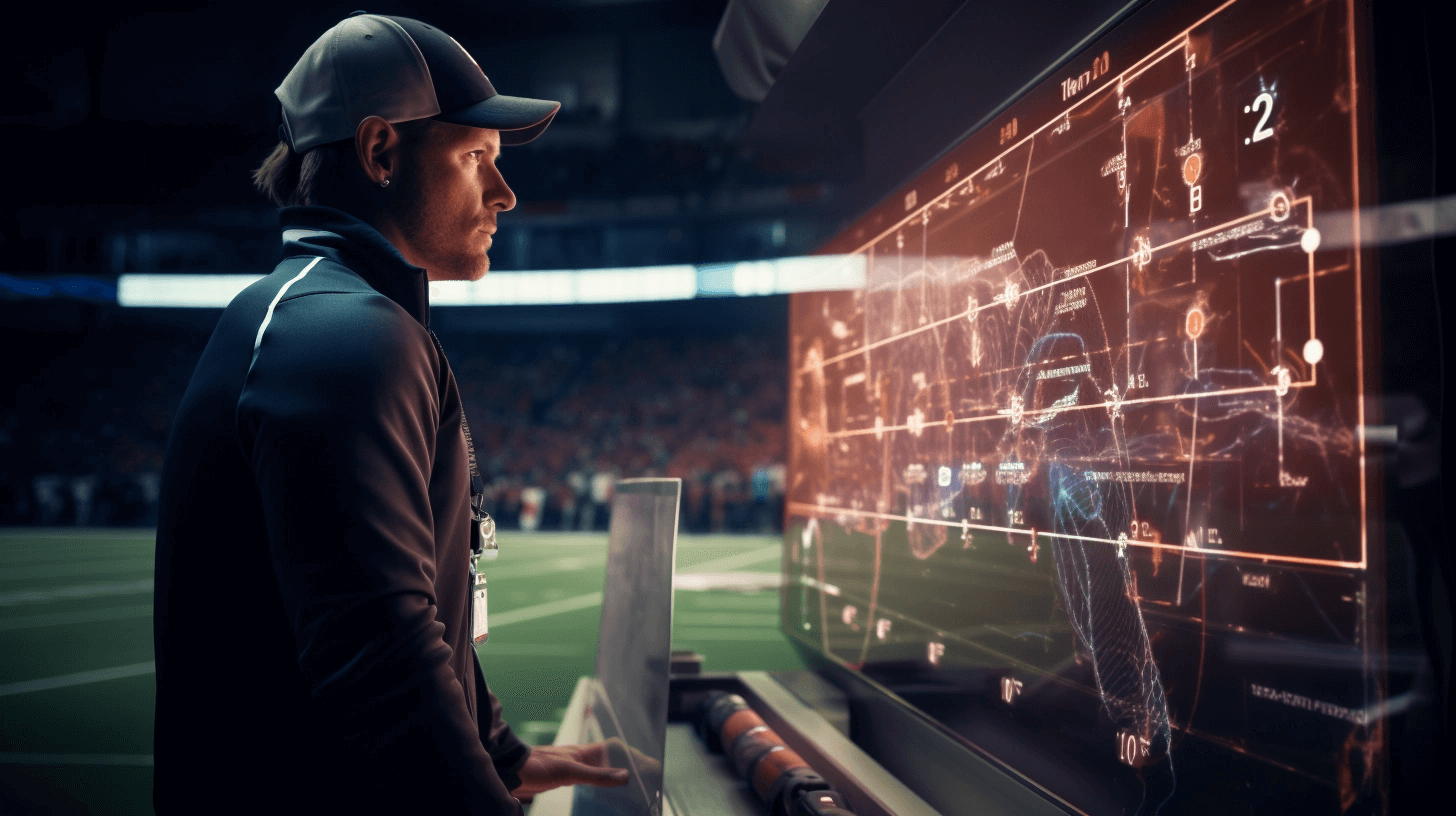
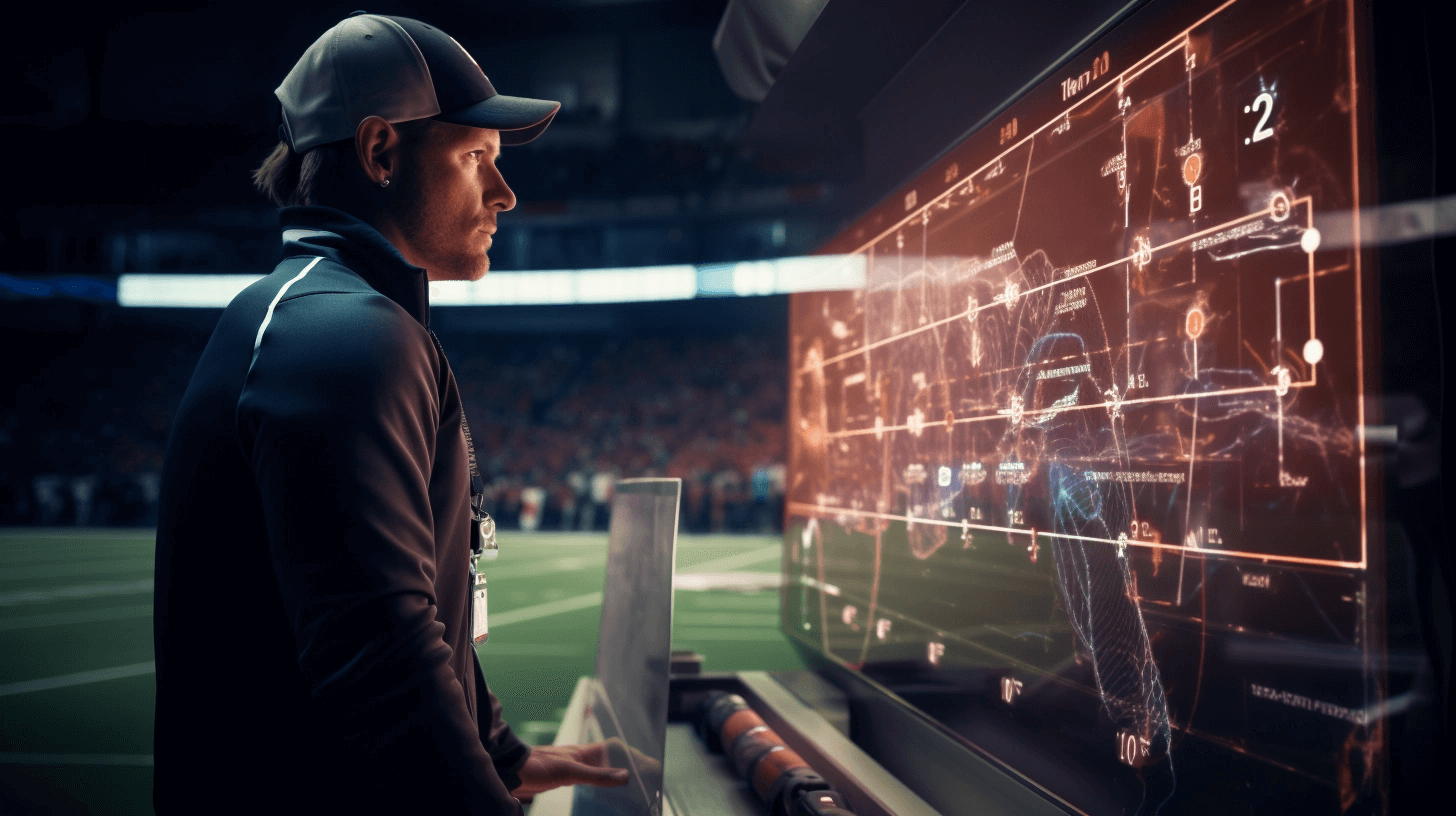
AI Sport Failures: Lessons for Sports Leaders
3 Min Read.
Biggest AI flops in 2024—it’s pretty wild!
In today’s fast-paced sports industry, artificial intelligence (AI) is no longer a futuristic concept—it’s a foundational tool. However, its meteoric rise hasn’t been without setbacks.
Over the past year, several high-profile AI failures in sports have illuminated the challenges of implementing these technologies effectively. Yet, every failure carries a silver lining: an opportunity to learn, adapt, and grow.
Below, we examine 12 notable AI missteps in the sports world and extract actionable lessons to help sports leaders navigate AI’s potential pitfalls and seize its transformative power responsibly.
AI Failures in Sports: 12 Stories, 12 Lessons
1. Sports Illustrated and the AI Author Backlash
Sports Illustrated faced public outrage after it was discovered that several articles were AI-generated but credited to fake authors with fabricated headshots. Readers felt deceived, leading to widespread criticism and loss of trust in the brand.
Lesson: Transparency builds trust. Clearly communicate when and how AI is used to avoid eroding stakeholder confidence.
2. The Klay Thompson Incident
An AI chatbot misinterpreted basketball slang, falsely accusing NBA star Klay Thompson of vandalism. The chatbot flagged the term “throwing bricks” (a slang phrase for missing shots) as vandalism, sparking a PR disaster.
Lesson: Train AI in industry-specific contexts to ensure cultural and technical accuracy.
3. Premier League’s Offside Tech Delays
The Premier League’s rollout of semi-automated offside technology in the 2023–2024 season faced delays due to inaccuracies when identifying offside positions in crowded penalty areas. Matches like Manchester City vs. Liverpool saw contentious decisions that ignited fan outrage and led to questions about the tech’s readiness.
Lesson: Rigorous testing in high-pressure environments is non-negotiable before deployment.
4. Michael Schumacher’s AI-Generated “Interview”
In April, a German magazine published a fabricated AI-generated interview with F1 legend Michael Schumacher, who has been out of the public eye since a 2013 accident. The article presented AI-crafted responses as if they were authentic, deceiving readers and sparking massive backlash from Schumacher’s family.
Lesson: Ethics and authenticity must guide AI-driven storytelling and fan engagement initiatives.
5. March Madness Failed Predictions
During March Madness, several AI-based sports betting tools failed spectacularly. These platforms, which promised predictive accuracy, overlooked critical human factors like momentum shifts and team psychology, resulting in poor betting advice. Bettors vented their frustrations online, eroding trust in AI’s predictive capabilities for high-stakes events.
Lesson: Use AI predictions as supplementary tools—not definitive answers—in decision-making.
6. Robo-Ump Errors in Baseball
In July, Major League Baseball tested automated umpiring systems in several games. During a high-stakes game between the Yankees and Red Sox, a crucial game-winning strikeout call was overturned by the AI system, which misjudged the strike zone. Fans and players debated whether the tech was ready to replace human umpires.
Lesson: Human oversight is crucial to balance AI’s precision with situational judgment.
7. NFL’s Missteps in Injury Prediction
The NFL’s “Digital Athlete” program aimed to identify high-risk players for injuries using AI. However, earlier this year it flagged several healthy players while failing to detect injuries in others, leading to criticism of the data quality.
Lesson: Data quality is king. Without accurate, diverse datasets, AI’s predictive power diminishes.
8. AI Misjudging Gymnastics Scores
At gymnastics competitions in 2024, AI-based scoring systems miscalculated performances, resulting in incorrect medal placements. Fans and athletes called the system “unfair,” prompting the governing body to revise the results.
Lesson: Combine AI with human expertise to maintain reliability in subjective assessments.
9. Goal-Line Technology Blunders
During a Premier League match between Aston Villa and Sheffield United, goal-line technology failed to detect a clear goal due to a rare camera occlusion. The incident directly impacted the outcome of the game, fueling debates about the reliability of AI in critical match situations.
Lesson: Always have redundancy systems and fallback mechanisms for critical AI tools.
10. Controversial VAR Calls at FIFA World Cup
AI-driven Video Assistant Referee (VAR) systems sparked controversy at the FIFA World Cup due to opaque decision-making processes. Fans and analysts criticized the lack of transparency in offside calls.
Lesson: Transparency in AI decision-making processes fosters trust among stakeholders.
11. Formula 1 AI Strategy Failures
Red Bull Racing’s reliance on AI to optimize race strategy backfired during the Monaco Grand Prix. The AI miscalculated tire degradation, leading to an ill-timed pit stop that cost the team the race. Over-reliance on AI without real-time adaptability cost teams crucial points.
Lesson: Real-time adaptability and human validation are vital in dynamic environments.
12. ESPN’s AI-Generated Sports Recaps Fall Flat
ESPN began publishing AI-generated recaps for women’s soccer and lacrosse games. These articles, labeled as authored by “ESPN Generative AI Services,” were intended to augment human journalism by covering less-publicized sports. However, the recaps were criticized for their blandness and lack of nuance, notably omitting significant events such as a star player’s final game.
Lesson: AI can assist in content generation, but human oversight is crucial to ensure depth, context, and emotional resonance in storytelling.
6 Critical AI Lessons for Sports Leaders
As AI reshapes the sports industry, its potential must be approached with cautious optimism. Here are six guiding principles for integrating AI responsibly:
- Prioritize Transparency: Clearly articulate AI’s role in processes to build trust among fans, players, and stakeholders.
- Invest in Testing: Pilot new technologies in controlled scenarios to refine performance before live deployment.
- Foster Collaboration: Use AI to enhance, not replace, human expertise in areas like refereeing and talent scouting.
- Context is Critical: Train AI models on domain-specific data to ensure relevance and precision.
- Address Bias Head-On: Conduct regular audits to identify and eliminate algorithmic biases.
- Prepare Backup Plans: Establish robust contingencies to handle technology failures in high-stakes environments.
Conclusion: Innovation Through Resilience
These stories of AI missteps in sports are not tales of failure—they are blueprints for progress. For sports leaders, these lessons underline the importance of strategic implementation and ethical considerations. AI’s role in sports is only set to grow, from elevating fan engagement to optimizing operations.
By learning from past challenges, leaders can ensure AI becomes a cornerstone of sustainable innovation rather than a source of recurring setbacks. The future of AI in sports is bright. With the right balance of ambition and caution, today’s failures can transform into tomorrow’s victories. Let’s lead the AI revolution in sports—not just by adopting technology but by mastering it.
Moving Forward – Personal Invite:
Looking to explore how AI can elevate your sports organization? Join our upcoming showcase session on Jan 15th, 2025 and discover the tools to stay ahead of the game.
With love for sports and Innovation,
Comments